Orbital-angular-momentum-encoded diffractive networks for object classification tasks
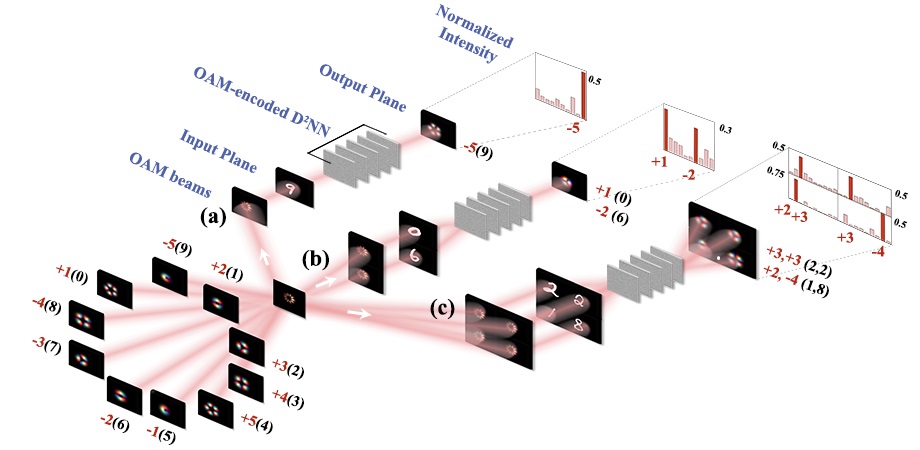
Deep learning has revolutionized the way we perceive and utilize data. However, as datasets grow and computational demands increase, we need more efficient ways to handle, store, and process data. In this regard, optical computing is seen as the next frontier of computing technology. Rather than using electronic signals, optical computing relies on the properties of light waves, such as wavelength and polarization, to store and process data.
Diffractive deep neural networks (D2NN) utilize various properties of light waves to perform tasks like image and object recognition. Such networks consist of two-dimensional pixel arrays as diffractive layers. Each pixel serves as an adjustable parameter that affects the properties of light waves passing through it. This unique design enables the networks to perform computational tasks by manipulating information held in light waves. So far, D2NNs have leveraged properties of light waves such as intensity, phase, polarization, and wavelength.
Now, in a study published in the Gold Open Access journal Advanced Photonics Nexus, researchers from Minzu University of China, Peking University, and Shanxi University in China have developed three D2NNs with diffractive layers that can recognize objects using information held in orbital angular momentum (OAM) of light. These include single detector OAM-encoded D2NNs for single and multitask classification, and multidetector OAM-encoded D2NN for repeatable multitask classification.
But what is OAM? It is a property of light waves related to its rotation or twisting motion. It can take on an infinite number of independent values, each corresponding to a different mode of light. Due to its wide range of possible states or modes, OAM can carry spatial information such as an object’s position, arrangement, or structure. In the proposed D2NN framework, OAM beams containing information illuminating handwritten digits are combined into a single vortex beam. This beam, containing multiple OAM modes, each associated with a specific twisting or rotation of light waves, passes through five diffractive layers trained to recognize the characteristics of handwritten digits from the OAM modes.
A notable feature of the OAM-encoded D2NN is its ability to discern the sequence of repeating digits. To achieve this, the researchers employed multiple detectors to process OAM information of multiple images simultaneously.
When tested on the MNIST dataset, a commonly used dataset for handwritten digit recognition, the D2NN correctly predicted single digits in the images about 85.49% of the time, a level of accuracy comparable to D2NN models that leverage wavelength and polarization properties of light.
Utilizing OAM modes to encode information is a significant step towards advancing parallel processing capabilities and will benefit applications requiring real-time processing, such as image recognition or data-intensive tasks.
In effect, this work achieves a breakthrough in parallel classification by utilizing the OAM degree of freedom, surpassing other existing D2NN designs. Notably, OAM-encoded D2NNs provide a powerful framework to further improve the capability of all-optical parallel classification and OAM-based machine vision tasks and are expected to open promising research directions for D2NN.
For details, see the original article by Zhang, Liao, et al., “Advanced all-optical classification using orbital-angular-momentum-encoded diffractive networks,” Adv. Photon. Nexus 2(6) 066006 (2023), doi 10.1117/1.APN.2.6.066006
Enjoy this article? Get similar news in your inbox |
|